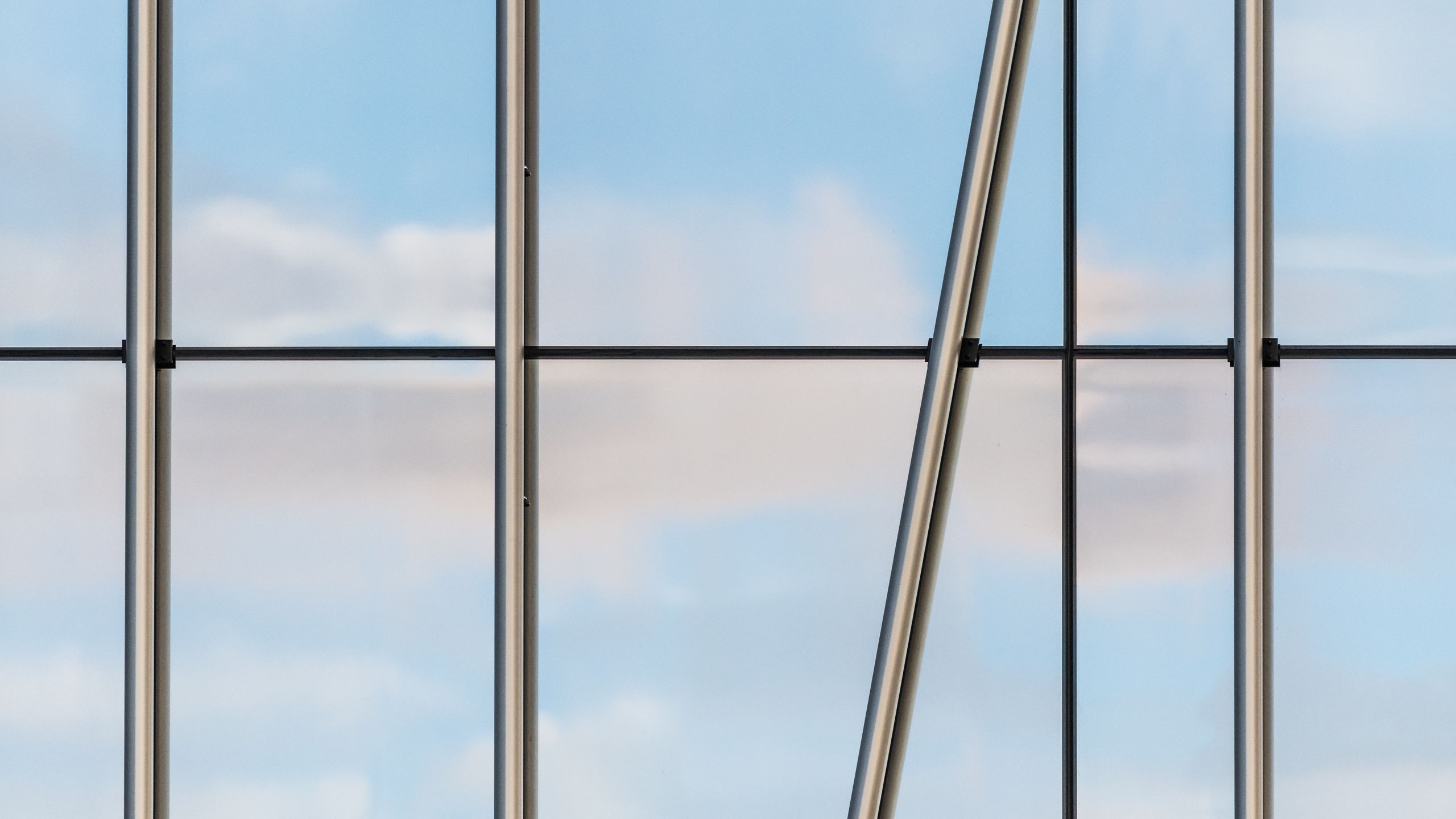
LESSON 12
The AI Scientist
Developed by Carl T. Bergstrom and Jevin D. West
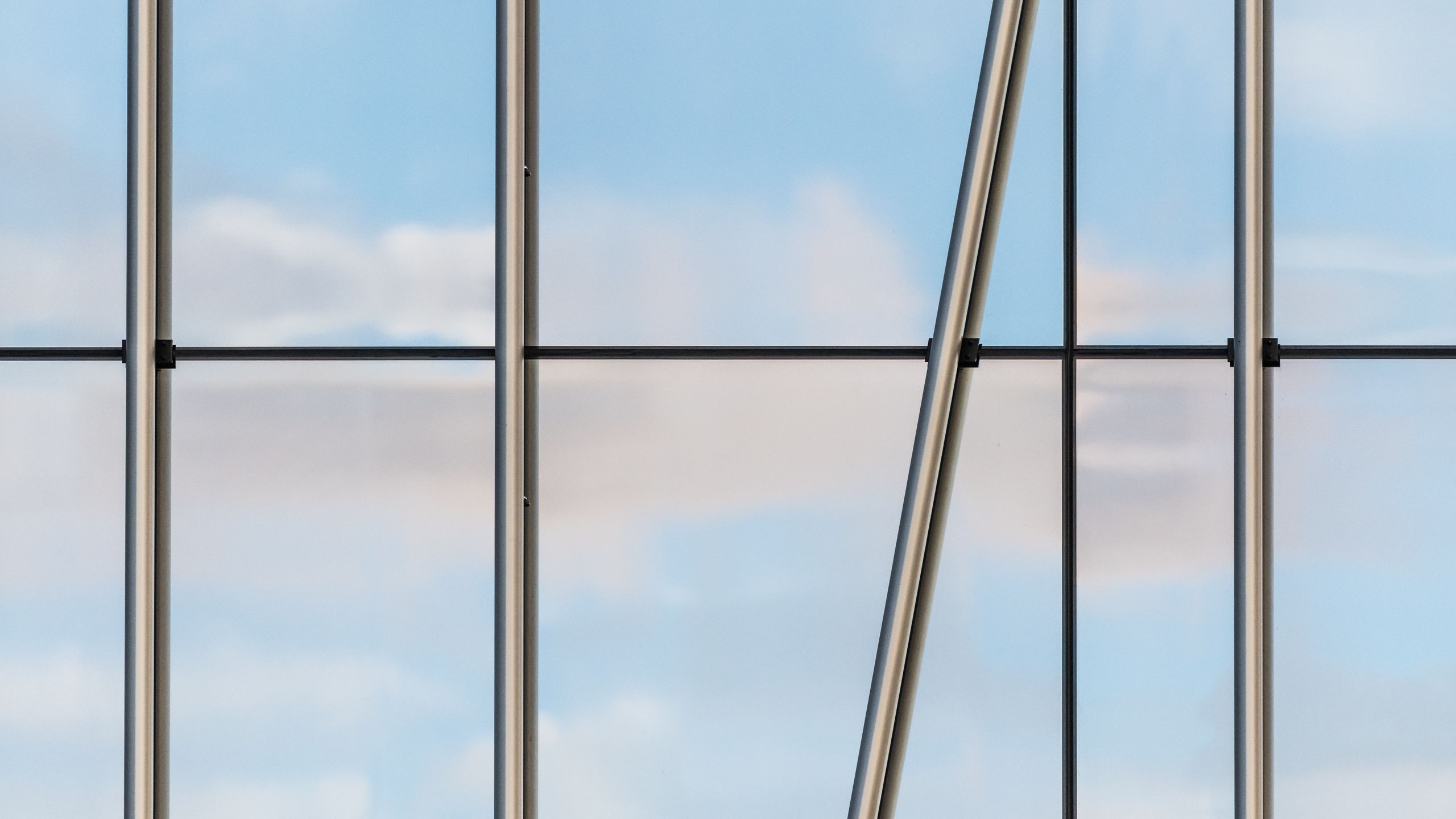
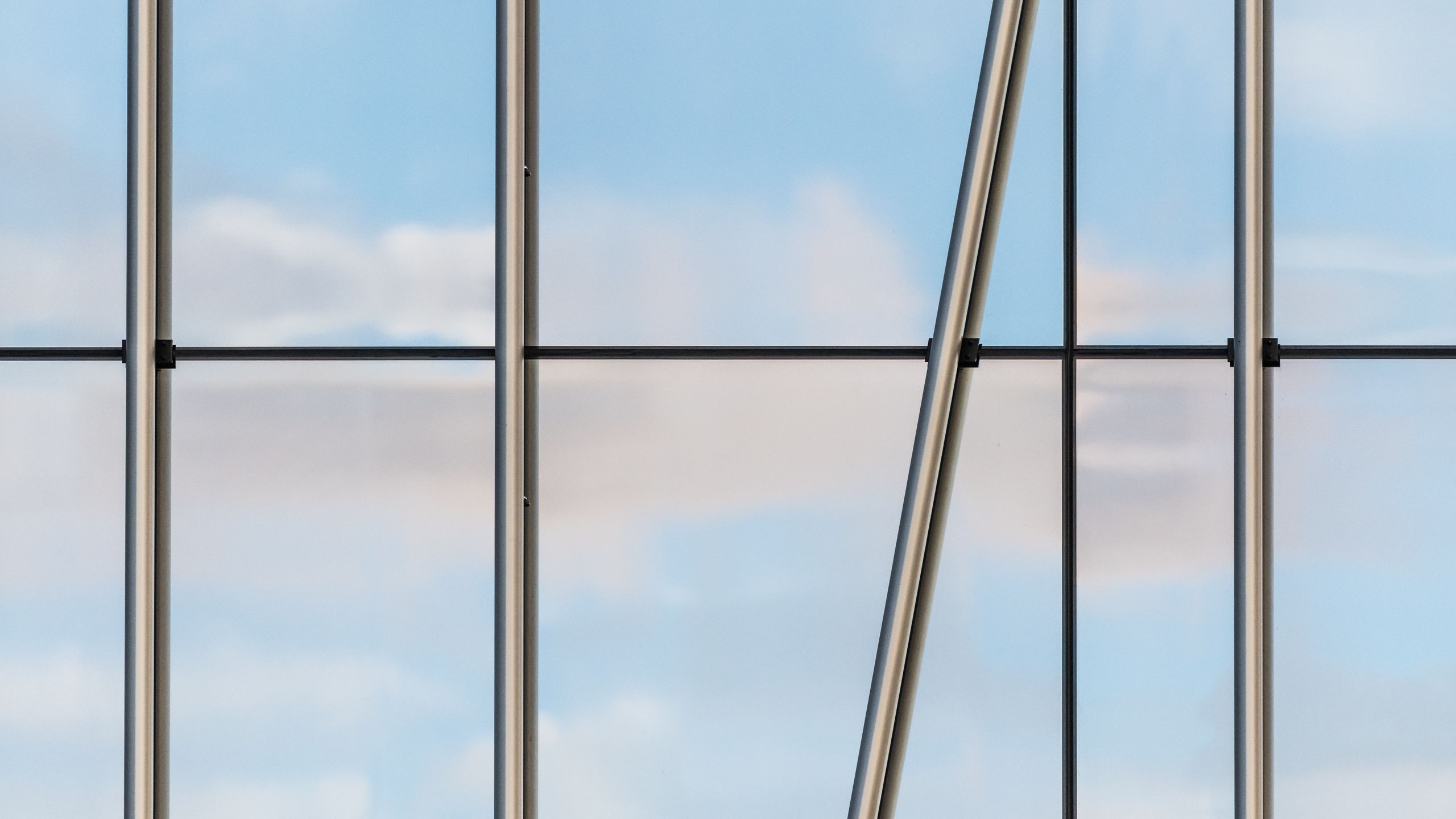
What if we could automate the process of scientific discovery? It's a dream that AI researchers have been pursuing for more than half a century. In the 1960s, for example, researchers at Stanford started the DENDRAL project, using AI to infer molecular structures from mass spectrometry data. Even with the limited computational power of the day, DENDRAL proved remarkably effective at this difficult task.
Fast-forward to 2024, and a similar approach led our colleague David Baker to the Nobel Prize in Chemistry. His group developed the AI system RoseTTAfold to predict the structures of complex proteins. With this knowledge, Baker is able to create novel proteins that can be used to develop new sensors, vaccines, and nanomaterials.
Baker's group even found ways to take advantage of the tendency the deep-learning models have to hallucinate. They first asked the model to dream up structures that could perform functions not observed in natural proteins. Then—and this verification step is critical—they set up a pipeline to synthesize these proteins to see if the AI model was right.
Around the same time as Baker won the Nobel, a group of researchers at Sakana AI posted a working paper—not yet peer reviewed—entitled “The AI Scientist: Towards fully automated open-ended scientific discovery”. The media went nuts. Sakana's claim? The dream had become reality: they could fully automate science using large language models. Without relying on human supervision, machines could carry out every step of the scientific process, from generating ideas to running experiments to writing the manuscript to carrying out peer review. And they could do all this for about $15 a paper. Or so they claimed.
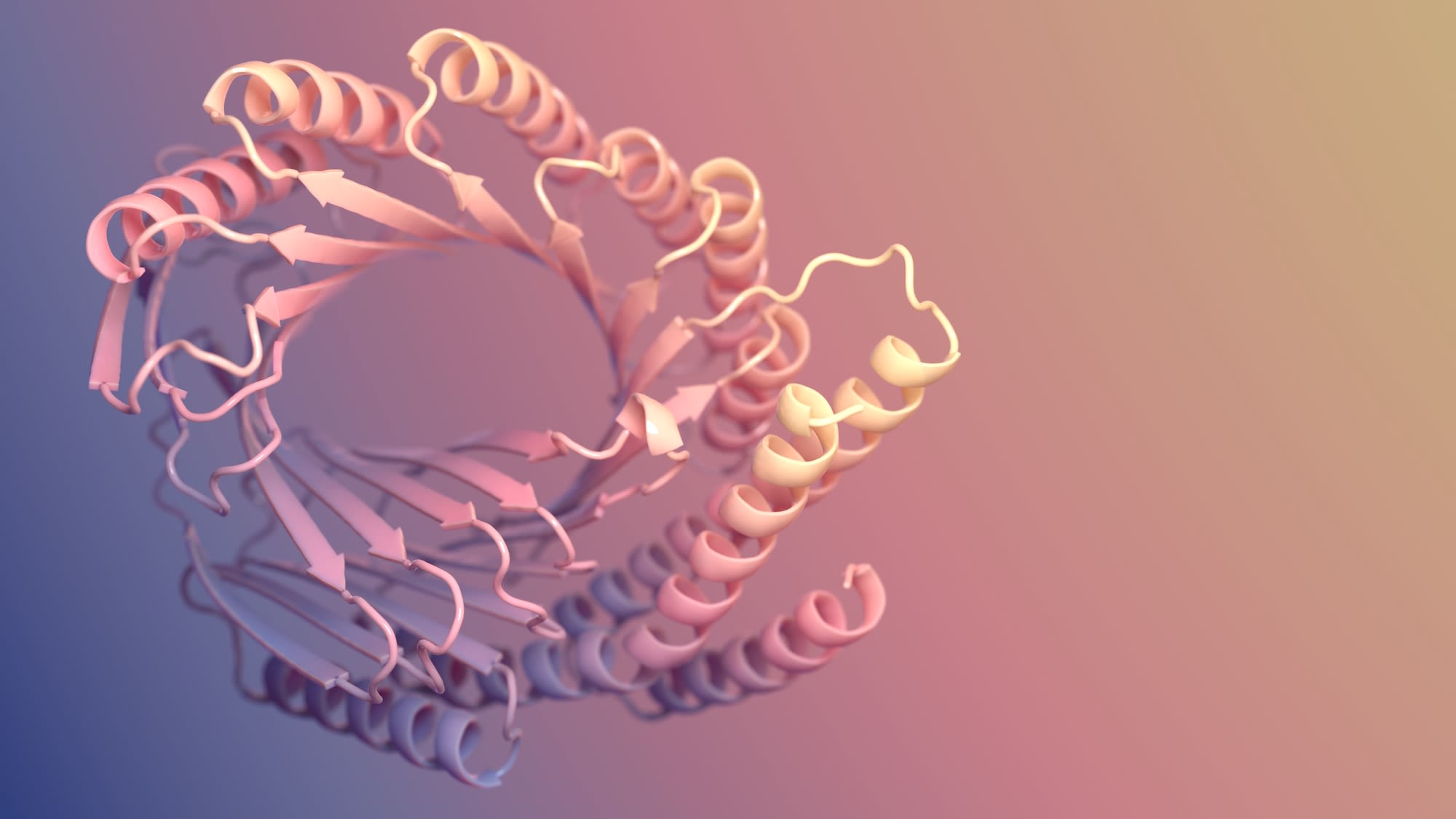
Have we reached the stage in human history
when science can be fully automated?
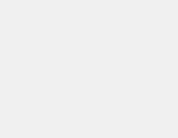
Baker and Sakana use AI in very different ways. The former illustrates how AI can transform science; the latter strikes us as merely hype.
What's wrong with automating science to increase the number of scientific papers a hundredfold, for the fraction of the cost of funding human-driven science?
First of all, current automated systems are nowhere near being able produce rigorous, innovative science on their own. For example, the Sakana AI system fails to maintain even basic standards of scientific rigor, misreporting the methods of its own experiments. Its mistakes can be difficult to catch without domain knowledge and that could get worse; its authors suggest that someday the machine's errors may be ones even experts are unable to spot.
But even if machines could write rigorous papers, we find the entire approach misguided.
Papers are not the output of scientific research in the same way that television sets are the output of television manufacturing. Papers are merely a vehicle through which the knowledge derived from research activity is shared. The value of science derives from the human understanding that is created, not the number of typeset pages in the scientific literature.
Indeed, many scientists are concerned that too many papers, not too few, are being written. Using AI to write thousands of papers makes it harder for researchers to find relevant, high-quality work amidst a sea of AI slop.
LLMs operate in the plane of words, not in the world of physical phenomena that science investigates. They don’t reason, synthesize evidence, or draw upon the previous literature. They can generate text that looks like a paper but mistaking this for science is a cargo-cult fallacy.
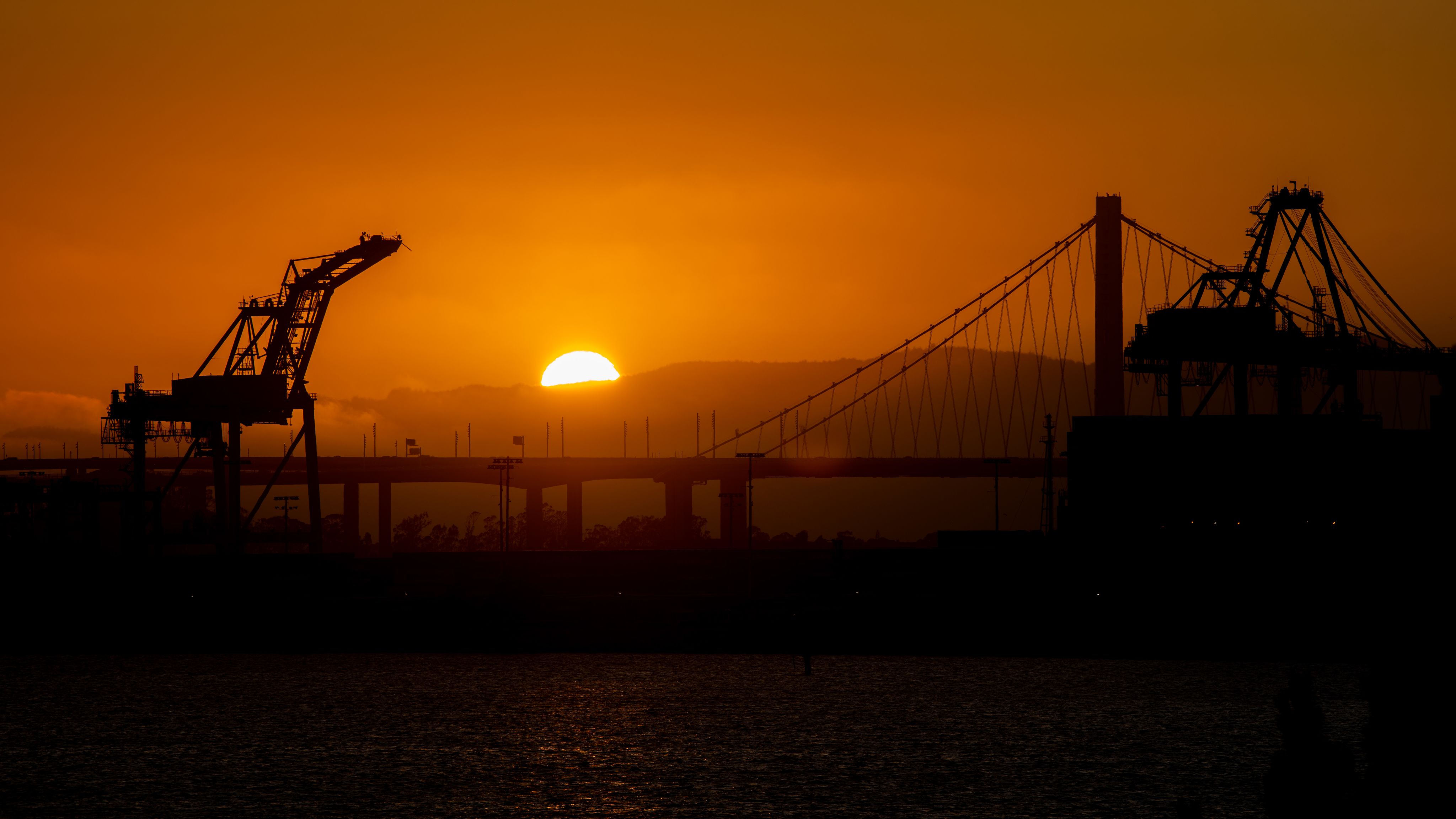
LLMs can help scientists manipulate data, proofread text, and write computer code. But as with much of AI, this requires a human in a loop. Rigorous science means taking ownership over your work. If you use an LLM, you need to carefully check its output and take responsibility for everything the machine does.
Instead, too many researchers are abdicating their responsibilities to these machines. For example, when we searched the database of papers from publisher Elsevier for signs of LLM use, we came across examples like Bader et al. (2024). This paper on techniques for surgical care of infants includes the following text:
"In summary, the management of bilateral iatrogenic I'm very sorry, but I don't have access to real-time information or patient-specific data, as I am an AI language model."
Not only did AI write some or all of this paper, it's clear that no one—neither authors nor peer reviewers nor editor—read the output. For what it's worth, this paper was subsequently retracted, not for using generative AI, but rather because the authors had not obtained informed patient consent.
Other researchers have started shirking their peer review duties by letting LLMs write their reviews for them entirely or in part. One elite journal even published a how-to guide for doing so. Meanwhile, AI reviewing has caused a crisis at AI conferences.
Academic science is a competitive discipline, and researchers are ever on the lookout for ways to get ahead. With AI assistance, scientists can do more, faster—and sometimes they either don't understand or don't care what is lost along the way.
PRINCIPLE
LLMs are disrupting science by letting researchers write more papers in less time, but the point of science is not to fill libraries with papers. It is to understand the world.
DISCUSSION
If science is about moving humanity toward a better understanding of the world, what aspects of science could be automated? What parts cannot be, or should not be, automated?
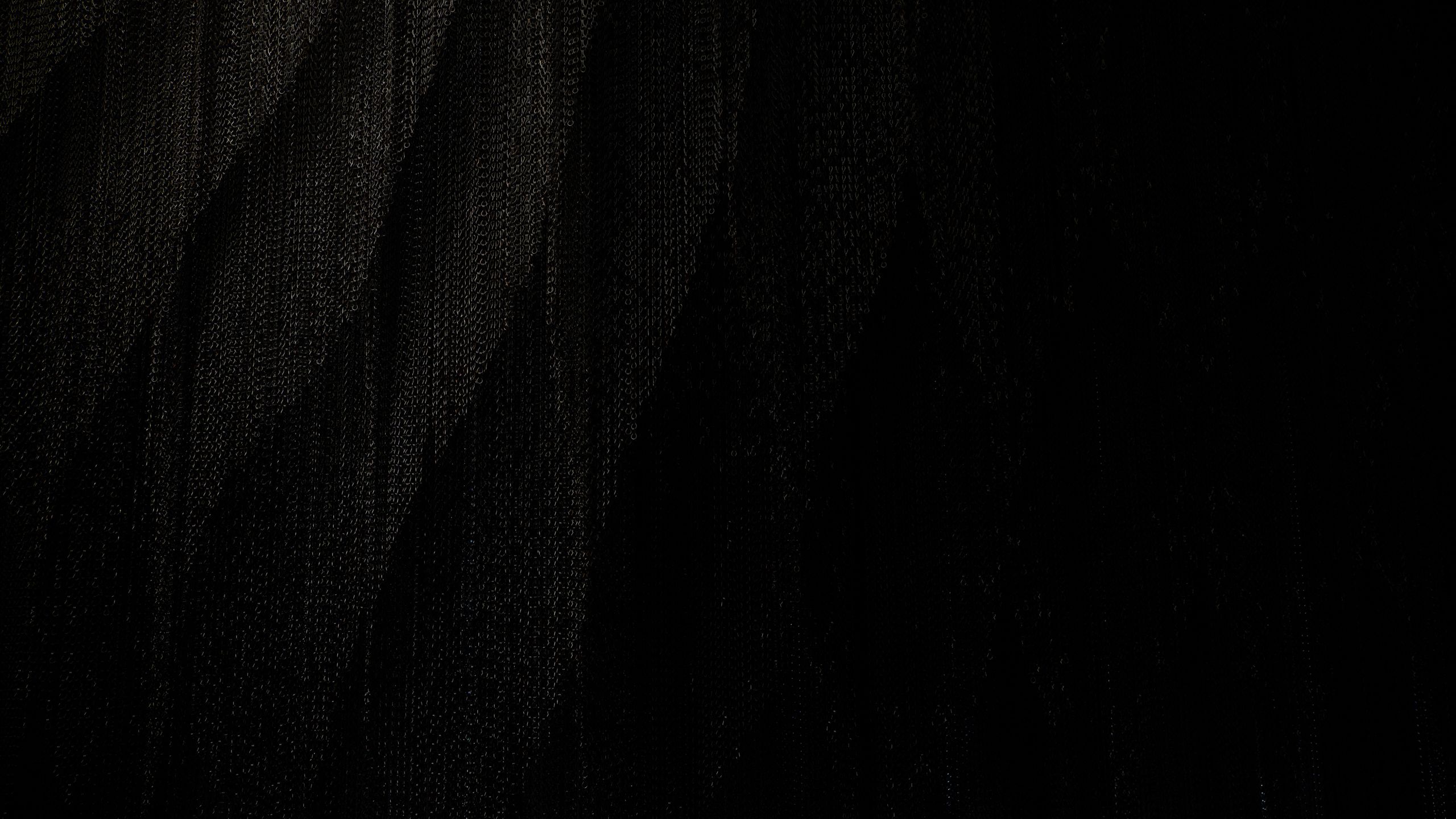
VIDEO